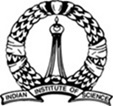 |
Modelling urban dynamics in rapidly urbanising Indian cities
a Energy & Wetlands Research Group, Center for Ecological Sciences [CES], Indian Institute of Science, Bangalore, Karnataka 560 012, India b Centre for Sustainable Technologies (astra), Indian Institute of Science, Bangalore, Karnataka 560 012, India c Centre for infrastructure, Sustainable Transportation and Urban Planning [CiSTUP], Indian Institute of Science, Bangalore, Karnataka 560 012, India d RCGSIDM, IIT Kharagpur, Kharagpur 721302, India
| 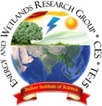 |
|
Introduction
Rapid urbanisation is a pivotal factor in altering the landscape structure affecting the ecology and environment during the last two decades (Sivaramakrishnan et al., 2005; MOUD, 2011; Ramachandra et al., 2012; Bharath et al., 2012; Ramachandra et al., 2014a). Urbanisation refers to a form of paved surface growth in response to increasing human activities with implications of economic, social, and political forces and to the physical geography of an area (Sudhira et al., 2007; Ramachandra et al., 2014a). The transformation of a landscape from rural to urban has a finite cycle with the regions evolving to form industrially dominant regions.
This would, further leads to rural push and spreading of city towards outskirts (Ramachandra et al., 2013). Dispersed urban growth in peri-urban regions is referred as urban sprawl. Sprawl takes place at the urban fringes in the form of radial development or development along the highways with the elongated urban development (Sudhira et al., 2003; Ramachandra et al., 2012). Regions under the influence of dispersed growth or sprawl are often devoid of basic infrastructure and amenities such as treated water supply, electricity, sanitation, etc. as planners were earlier unable to visualise these sporadic growth pockets. This has necessitated studies on urban sprawl across the globe (Batty et al., 1999; Torrens, 2000; Sudhira et al., 2004; Huang et al., 2007; Bhatta, 2009a,b, 2010; Ramachandra et al., 2012, 2015). Often these sprawled regions are left out in the in population census and also in vision documents such as city developmental plans, etc. However, technological advancements in remote sensing technologies and Geoinformatics (Geographic Information system (GIS)) hasovercome the limitations of data, skills, etc. (Adhvaryu, 2011; Bharath et al., 2014).
Remote Sensing data acquired through space borne remote sensors enables a repetitive synaptic bird eye view of the landscape at low cost (Lillesand and Kiefer, 2005). Availability of multiresolutions (spectral and temporal) spatial data at local, regional and global scales helps in land cover (LC), Land use (LU), land use land cover changes (LULCC) and surveillance of problematic sites (Campbell, 2002). Spatial data acquired remotely aids in the identification and assessment of land use patterns which is important for environmental management and decision making. Availability of these data at regular intervals have aided in advance simulation of urban growth for planning of infrastructure and basic amenities. Simulation of land use would provide an excellent feedback to plan and understand the real world scenarios and changes before implementing on ground (Zhang et al., 2011). Thus defined as a process of changing scenarios variables and observing the results to study the levels of abstraction in changing scenarios and change of events (Banks et al., 2004). Simulation also allows planners and city managers to study a problem at several different levels of abstraction. By this a planner can understand the complexity of the overall system (Santé et al., 2010). Early 90’s urban simulation approaches were based on theories, and suffered from significant weaknesses due to lack of understanding of space–time dynamics. The integration of space, time, and attributes in modelling was further enhanced with the evolution of Cellular automata (CA) models (Allen, 1997; Batty et al., 1999; EPA, 2000; Alberti and Waddell, 2000). CA techniques are simple and easily integrated with raster GIS with adaptability to various urban growth situations. CA models represent complex patterns through the use of simple rules and considering its neighbouring properties since these models operate on basis of cell states, size, neighbourhood and transition rules (White and Engelen, 2000). CA modelling aids in addressing the spatial complexity with discrete time changes, evident from satisfactory simulations of spatial urban expansions (Clarke et al., 1997; Leao et al., 2004; Bharath et al., 2013; Ramachandra et al., 2013; Arsanjani et al., 2013). Advantages of CAs are based on sitespecific rules that are predefined based on historical transitions represented by pixel based raster simulations in discrete time (Guan et al., 2011). CA based urban models usually pay more attention to simulating the process of urban development and defining the factors or rules driving the development (Batty, 2007). The CA model with powerful spatial computing can be used to simulate the spatial variation of the system effectively. CAs represent local raster-based simulation for modelling urban expansion for discrete time steps (Guan et al., 2011). Despite these appealing properties, CA models lack the ability to account for the actual amount of change. Therefore, coupling the MC and CA approaches (Eastman, 2009) provides a powerful modelling framework in which the shortcomings of each are eliminated. A The CA–Markov model absorbs the benefits from the time series and spatial predictions of the Markov and CA theory, and it can be used to carry out the Spatial–Temporal Pattern stimulation. The CA–Markov model also considers the land use changes’ suitability and the effect of natural, societal and economic factors about land use changes. Different CA models have been developed to simulate urban growth and urban land use/cover change over time. The differences among various models exist in modifying the five basic elements of CA, i.e., the spatial tessellation of cells, states of cells, neighbourhood, transition rules, and time (White and Engelen, 2000; Liu, 2009). Among various others approached such as regression modelling have been effectively analysed and applied (Arsanjani et al., 2013).
1.1. Agent based modelling framework
Urban growth is mainly altering the land use changes according to human activities with complexity involving drivers that are influenced by human thinking and natural drivers (Le et al., 2008). Land use change occurs from decisions made by individual drivers that are derived from various socio economic ecological setting. These changes are rather complex and needs understanding by approaches that are process oriented, pattern oriented or objects of change oriented (CO) associated to human and natural drivers. Considering these CO process would help in help in understanding micro to macro level process and in policy interventions affect the behaviour of the individuals that produce changes at micro-level (Lambin et al., 2003). These non-linear transformative processes are not captured using normal conventional models (Leet al., 2008). Land-use models that are statistically driven often ignore human drivers as transformation of land use factors (Veldkamp and Verburg, 2004). Agent based modelling has been recognised to be well suited to express the co-evolution of the human and landscape systems based on the interactions between human actors and their environment (Batty, 2001; Verburg et al., 2005). This essentially requires to understand the process of modelling requires integration of human drivers socio economic drivers and classic land use change models. The objects of change oriented models are therefore useful in integrating these drivers using real land use change altering forms through understanding the behaviours of various known entities that are influential in attracting the urban growth. These influential agents are termed as ‘‘Agents of change” (Castella and Verburg, 2007). The coupled human, socio–economic, environment system is described in the form of agents which have their specific roles in modelling land use change. Agents are described by several characteristics they are self-governing, interacting systems and behave with mutual effect to environment. Agents have been used to represent a wide variety of entities, including industries, cars, people, cells, social factors etc., (Parker et al., 2003; Robinson et al., 2007; Wooldridge, 2009). Thus, the agents can represent human behaviour more accurately than the conventional models (Fujita and Kashiwadani, 1989). Jokar et al. (2011) used a CA-Markov model to monitor land use changes and to predict future states. Further Arsanjani et al., in 2013 showcased employed a logistic regression based CA model integrating agents but could not attribute the behaviours of agents to illustrate human tendency of being fuzzy in nature. Artificial Neural Networks (ANN) was also employed by Tayyebi et al. (2011) to develop an agent based model but this could integrate human tendency but failed to exhibit the real complex behaviour of agents in urban systems. There has been an development of various Multi agent simulation based systems but these have not showcased the effectiveness of mapping policy, social, economic data with human behaviour and actions. Therefore, the primary objective of this study is to consider the behaviour and the preferences of humans, agents (which represents the examples of social, economic, policy decisions) and to model urban growth in five major metropolitan areas of India. This has been accomplished by integration of CA Markov with AHP (Analytical hierarchal process) and Fuzzy for providing multi criteria performance with the definitive spatial allocation of changes (Eastman, 2009, Jokar Arsanjani et al., 2013) and to integrate the behavioural contribution. Artificial intelligence based fuzzy analysis coupled with Analytical hierarchal process (AHP) that integrates agents is used in the current study to account for changes in the allocation pattern in rapidly urbanising five landscapes in India. Fuzzy and AHP were used to characterise the agent’s behaviour and to compute the influence of agent(s) on land use and urban growth. and urban growth with spatio temporal observations of neighbourhood change was computed with CA-Markov based analysis. Thus integrating agents with conventional modelling system.
Fuzzy transition rules: Urbanisation process is complex involving multiple agents with diverse patterns of behaviour under changing spatial and temporal scales (Cheng and Masser, 2003), while mimicing the dynamic process of urbanisation. In recent
years the integration of Cellular Automata with artificial intelligence techniques (such as neural networks, etc.). Fuzzy logic gave better performance than statistical techniques (Yeh and Li, 2002; Guan and Clarke, 2005; Mandelas et al., 2007; Pradhan and Pirasteh, 2010; Janssen et al., 2010; Keshavarzi and Heidari, 2010). Fuzzy logic also integrates modelling human behaviour of thinking and model complex environments (Han et al., 2005). Fuzzy rules specifically are built upon a set of fuzzy expressions which evaluates every specific attribute of the function. Fuzzy rule can provide a better of modelling output that simulates the real world situation through different function and can have different membership degrees to the allocation pixels (Dubois et al., 2007). The final decision is reached with maximising the membership degree. Urban models have been developed (Mantelas et al., 2008) through a set of fuzzy rules to process the data and calculation of indices regarding urban pattern of development and these indices are used as rules in a Cellular Automata model to predict urban growth.
Analytical Hierarchal Process (AHP): AHP has been used (de Quadros et al., 2006; Elaalem et al., 2010; Elaalem, 2013)as multi-criteria decision making (MCD) techniques with fuzzy rules and indices to weigh characteristics of diverse opinions in a complex environment. This involves choosing the criteria to measure the agent’s effectiveness in a landscape, specifying alternatives and assigning weights to the criteria of observation. AHP is commonly used MCD technique for suitability o⁄+-f landscape through pair wise analysis (Chang et al., 2008; Chen et al., 2010; Thapa and Murayama, 2008). Artificial intelligence integration with MCD techniques improves efficiency towards modelling real world situation for understanding spatial patterns of urbanisation. This study models the urban process through Cellular Automata with criteria and decisions from real world using different agents (of influences).
|
Citation : H.A. Bharath, M.C. Chandan, S. Vinay, T.V. Ramachandra, 2018. Modelling urban dynamics in rapidly urbanising Indian cities. The Egyptian Journal of Remote Sensing and Space Science, Volume 19, Issue 2, December 2016, Pages 175-193, https://doi.org/10.1016/j.ejrs.2016.09.001
* Corresponding author
H.A. Bharath
Ranbir and Chitra Gupta School of Infrastructure
Design and Management (RCGSIDM), Twin Science Block, IIT-Kharagpur, Kharagpur,
West Bengal 721302, India.
E-mail : bharathhaithal@gmail.com
|
|
|